Understanding S19A Status Requirements A Legal Analysis for AI Contract Assessment in Transportation Compliance
Understanding S19A Status Requirements A Legal Analysis for AI Contract Assessment in Transportation Compliance - Mandatory Technical Safety Protocols Under S19A Transportation Laws 2024
The recent amendments to S19A, specifically the "Mandatory Technical Safety Protocols," mark a shift in the regulatory landscape of transportation, particularly public transit. These protocols, a key component of the revised Public Transportation Agency Safety Plans (PTASPs), demand a comprehensive approach to safety from transit agencies. Essentially, agencies are now obligated to proactively manage safety risks, adopting strategies that demonstrably mitigate risks. This includes, notably, measures to ensure the safety of both transit workers and the public. Preventing incidents such as assaults on transit personnel and vehicle-pedestrian collisions are explicitly highlighted as areas needing rigorous attention.
The practical implication of these new protocols is that transit agencies must incorporate these safety measures into their operational procedures and compliance structures. While this shift signifies an increased emphasis on safety and ultimately, a safer environment for all involved, it also places a considerable burden on agencies to demonstrate adherence to these standards. The efficacy of these protocols remains to be seen, however the intention is clearly to move the needle on transportation safety and to create a more reliable and accountable framework for the future of public transit.
Following the enactment of the S19A Transportation Laws in 2024, a significant emphasis has been placed on integrating technology into vehicle safety. This is apparent in the requirement for all newly designed vehicles to include real-time monitoring systems for compliance with safety regulations, effectively shifting towards a more technological approach to ensuring vehicle safety.
Beyond the real-time monitoring, S19A mandates the integration of redundant braking systems into all vehicles. This is a sensible precaution intended to ensure that vehicles remain operational even if the primary braking system malfunctions, improving overall safety. However, the biannual audit mandate, where external reviewers verify compliance with these technical specifications, feels somewhat heavy-handed. It seems to be aimed at enforcing a rigorous standard of safety, but also potentially creates a burden on transportation companies.
The new laws also stipulate data-sharing capabilities in all new vehicles. This appears to be a direct attempt to enhance emergency response times in case of transportation accidents by allowing rapid data transfer to the relevant authorities. Though potentially beneficial, this raises questions about the volume and nature of data being transmitted, as well as privacy considerations for both passengers and vehicle operators.
Further, S19A imposes a minimum sensor accuracy threshold for automated systems. This push for increased sensor sophistication will likely lead to the development of even more advanced sensors, which could be a positive outcome. However, it's worth considering how the threshold was determined and whether it's the most efficient method for achieving the intended safety goals.
In addition, the legislation requires dedicated training for those operating autonomous vehicles. This training emphasizes not just the technical aspects, but also the ethical dilemmas that might arise in autonomous vehicle operation. It's a thoughtful approach to address the challenges of complex decision-making in autonomous vehicles, but questions remain about whether the training programs are adequately standardized and enforced.
The expanded scope of S19A extends even to the maintenance side of transportation with the mandate for certification processes for vehicle mechanics performing safety checks. While this does suggest an attempt to improve safety standards throughout the industry, it's interesting to consider if this increase in regulation may inadvertently create barriers to entry for smaller repair businesses.
Concerns surrounding cybersecurity are also being addressed through the inclusion of safeguards in S19A. The law necessitates that all vehicles include protections against cyberattacks that could endanger vehicle safety. This is a critical aspect, considering the vulnerabilities associated with increasingly connected vehicles, and emphasizes the importance of proactive security measures.
To ensure continual safety improvement, the law also promotes a comprehensive incident reporting system that demands a real-time analysis of any safety breaches. This structured reporting process should provide a valuable data resource for improving future safety regulations. It is however important to consider if this real-time analysis introduces excessive administrative burdens for transport operators.
Finally, S19A places specific restrictions on the use of unmanned vehicles in densely populated areas, which acknowledges that such situations can pose unique safety risks due to pedestrian density. This suggests a recognition of the need for contextually specific regulations for autonomous transportation. It remains to be seen, however, if these restrictions are flexible and adaptable enough to navigate the complexities of evolving urban environments.
Understanding S19A Status Requirements A Legal Analysis for AI Contract Assessment in Transportation Compliance - AI Risk Management Framework Updates Required by Federal Guidelines
Federal guidelines are increasingly emphasizing the need for robust AI risk management frameworks, particularly in the context of generative AI. The NIST AI Risk Management Framework, released in July 2024, includes a specific profile for generative AI to address the unique risks these technologies present. This push for AI risk management stems from President Biden's 2023 Executive Order, which prioritizes the creation of a secure and trustworthy AI environment built upon societal values and ethical considerations.
The framework promotes a human-centric approach to AI development and use, incorporating risk management into existing organizational processes. It's meant to make AI implementation more efficient and responsible. The OMB's subsequent guidance outlines minimum risk management practices for AI systems impacting individual rights and safety, a move that suggests a heightened focus on the ethical and societal implications of AI development, especially within federal procurement.
While these new frameworks offer a roadmap for organizations to manage AI risks, it’s important to consider how these requirements might impact various sectors, especially those with complex operational needs like transportation. The challenge becomes ensuring that the framework’s implementation doesn't become overly burdensome and hinders innovation or efficient operations. The balance between managing potential risks and encouraging responsible AI development within various industries will likely be a key consideration moving forward.
Following the Executive Order on AI from October 2023, the NIST released the AI Risk Management Framework (NIST AI 6001) in July 2024, with a specific focus on generative AI. This framework, building upon the earlier "Blueprint for an AI Bill of Rights," is aimed at fostering the development of safe, secure, and trustworthy AI systems. The OMB's implementation guidance further solidified this, outlining minimum risk management practices for AI applications influencing safety and human rights, especially in areas like public transit.
The core philosophy behind this framework is a human-centric approach to AI, prioritizing social responsibility and sustainability in the design and use of AI. It's intended to fit smoothly within existing organizational risk management procedures, making it more effective and seamless. Interestingly, even before the current version, a second draft was released in August 2022, inviting public input and accompanied by an AI RMF Playbook to assist in implementation. The framework itself promotes a risk-based, resource-conscious, and voluntary participation model, developed transparently and openly.
President Biden's Executive Order underlined the urgent need for standardized AI risk management within the federal procurement process. The core idea here is that AI decisions, especially in areas like transportation where safety is paramount, should align with societal values. This framework aims to help agencies operationalize this idea, by ensuring responsible AI practices during the development and implementation of AI-powered systems, including the integration of new technology into transportation vehicles.
It's important for organizations to regularly assess risks associated with AI, including identifying biases in the algorithms and their output. Transparency is also emphasized, requiring agencies to be upfront about the methodologies they employ, which is crucial for building public trust. Furthermore, the framework requires robust contingency planning, including regular testing, to ensure system resilience and operational continuity in the event of emergencies.
Another notable aspect is the need for inclusive decision-making, which requires agencies to gather diverse perspectives, not only from internal teams but also from passengers and other stakeholders. This creates a wider scope of input when deciding on safety-related matters. It also establishes stricter audit requirements, where organizations must be prepared for both announced and unannounced audits, not just focusing on current compliance but also assessing the effectiveness of their ongoing risk management procedures.
Ensuring cybersecurity has also been elevated in importance. The framework mandates regular penetration testing to defend against potential cyber threats, underscoring the necessity of continued investment in protecting AI systems utilized in critical infrastructure like transportation networks. The framework also recognizes that AI decisions can have far-reaching socio-economic implications. For example, the development and deployment of automated systems might not equally benefit different demographic groups, making it critical to assess these potential consequences.
There's a stronger emphasis on responsible data retention with the introduction of clear data storage policies. This helps balance the need for data to analyze safety issues with mitigating potential privacy concerns. The importance of ethical AI guidelines is also highlighted in the framework, forcing organizations to develop and follow these guidelines for decision-making in AI systems used in transportation.
Finally, the updates also stipulate training programs for all personnel who interact with AI systems, not only to ensure they're technically proficient but also that they understand the larger implications of those AI decisions. This is essential for aligning human judgment and oversight with automated systems, particularly in safety-critical fields.
While these updates signal a positive step towards responsible and safe AI implementation, it's important to remain critically aware of the potential consequences. The efficacy of these mandates, how they're implemented, and their impact on different stakeholders remains to be seen. Further research and assessment are critical to understanding how these changes will impact the safety and the broader societal context of transportation.
Understanding S19A Status Requirements A Legal Analysis for AI Contract Assessment in Transportation Compliance - Transportation Data Privacy Requirements in AI Contract Assessment
The growing use of AI in transportation brings about crucial questions regarding data privacy. Transportation systems increasingly rely on a vast amount of data generated by users and devices, creating a need to strictly adhere to privacy regulations like the GDPR. This means transportation agencies and those developing AI for transit applications need to thoroughly analyze how data is handled through privacy impact assessments. This process ensures compliance with legal requirements and promotes transparency about how data is used. Drafting effective contracts that cover a wide range of legal responsibilities for both AI developers and transportation providers is another challenge. This involves carefully considering the different laws that might apply to the providers and users of the AI systems. Ultimately, ensuring data privacy alongside the benefits that AI brings to the transportation industry is vital for maintaining public trust and a secure environment. Striking a balance between progress and protection is a core issue that will need careful consideration going forward.
The increasing use of AI in public transportation, while promising efficiency and accessibility, raises critical questions about data privacy and surveillance. Transportation authorities are now compelled to address these concerns through a tighter regulatory framework, particularly within S19A. The principle of data minimization is being emphasized, suggesting that only data absolutely necessary for safety and compliance should be collected. This is a step towards reducing the risk of potential misuse of sensitive passenger data.
Furthermore, anonymization techniques are being promoted to safeguard the identities of drivers and passengers. Even in the event of a data breach, robust anonymization would ideally prevent the exposure of personal details. Previously more flexible regulations regarding third-party data sharing have been replaced with strict limitations, aiming to protect individual privacy in collaborative arrangements.
Passengers now have more control over their data, as the regulations require transportation companies to implement clear opt-out mechanisms. This gives passengers the ability to choose whether they wish to participate in data collection practices. However, the integration of AI can introduce unforeseen challenges. For instance, automated fare systems or AI-driven risk assessments may lead to unintended profiling or discriminatory outcomes, so agencies need to analyze how these algorithms affect privacy.
To proactively address these concerns, S19A now mandates Privacy Impact Assessments (PIAs). This requirement pushes agencies to evaluate the privacy risks of any new technology before deployment. Likewise, the scope of real-time data usage is being limited to specific circumstances, particularly in emergency situations. This is aimed at reducing the potential for constant surveillance beyond necessary use cases.
Stronger security measures are being implemented, with a focus on encryption and secure access to safeguard sensitive information. In a bid to improve transparency and public trust, agencies are now obligated to create and release transparency reports detailing their data practices. This greater transparency encompasses data collection, retention, and sharing procedures.
Further, to enforce compliance, regular privacy audits will be conducted by independent bodies. These audits will ensure ongoing adherence to the new privacy standards and provide opportunities for agencies to identify and correct areas of deficiency. It's clear that S19A has shifted towards a much more cautious and stricter approach to data privacy in the transportation sector. While the intent is admirable, the ongoing impact on innovation and operational efficiency in transportation remains to be seen. Additionally, it will be interesting to observe how effective the audit process will be in ensuring long-term compliance with these evolving privacy standards in the dynamic environment of AI-powered transportation systems.
Understanding S19A Status Requirements A Legal Analysis for AI Contract Assessment in Transportation Compliance - Evidence Based Training Standards for AI Systems in Contract Review
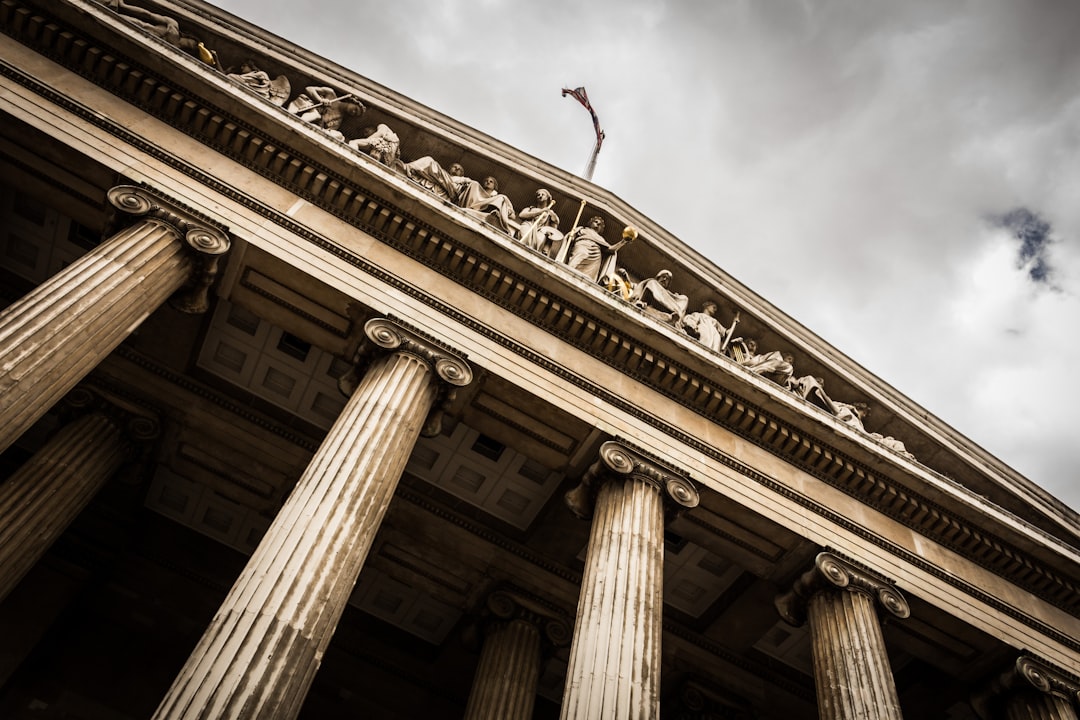
The development of evidence-based training standards for AI in contract review represents a significant shift towards more reliable and effective AI use in legal settings. These standards promote a structured approach to training AI, aiming to improve its ability to accurately understand and interpret legal language within contracts. This, in turn, can potentially minimize review errors and increase the efficiency of legal processes. With AI playing a growing role in supporting legal professionals, these rigorous standards become crucial for establishing confidence and ensuring AI-driven decisions are accountable.
However, it's vital to consider the potential impact these standards might have on the evolving legal landscape, especially in regards to finding the right balance between automated processes and human involvement. As the field continues to advance, organizations should be wary of potential downsides that might emerge from excessive reliance on AI in intricate legal environments. It's a delicate balancing act to ensure the benefits of AI are realized without undermining the importance of human expertise and judgment in areas where nuanced understanding of the law is critical.
AI systems are increasingly being used in contract review, particularly in areas like transportation where regulations are complex. The use of AI in this context can streamline processes, reduce human errors, and improve efficiency, especially when dealing with large volumes of contracts. However, ensuring these AI systems operate reliably and comply with regulations like S19A necessitates rigorous standards, especially in the training data used to develop them.
Evidence-based training standards for AI in contract review, if properly implemented, can help bridge this gap. They can significantly improve the compliance of AI systems with S19A's requirements. For example, by training AI on real-world transportation contract data that reflects the nuances of the industry, we can ensure the AI can handle the complexities of these contracts more effectively.
The call for thorough documentation within these standards is a key aspect. It means there's a clear audit trail, allowing us to trace back AI decisions to the specific data used in its training. This transparency can greatly improve accountability and potentially lessen the risk of legal challenges. Some research suggests that using evidence-based training can substantially reduce biases in AI outputs compared to more traditional training methods, leading to fairer and more equitable handling of different contract scenarios.
Incorporating diverse data inputs during AI training is crucial. This approach not only improves performance but also enhances an AI's ability to predict and mitigate potential legal disputes, since it's exposed to a wider range of contractual situations and their outcomes. It seems that using robust validation processes during training can greatly decrease error rates in AI contract review compared to human-led reviews. This accuracy can translate to more precise compliance with regulations and potentially minimize legal risks.
These evidence-based training standards also mandate regular assessments of how AI systems make decisions. This requires organizations to evaluate AI recommendations against established legal criteria. As AI training protocols are updated, it's essential to maintain a strong partnership between AI developers and legal experts. This feedback loop ensures the AI's understanding of transportation laws remains current and refined.
By meeting these evidence-based standards, companies can gain a competitive advantage. Organizations that successfully deploy these systems often see smoother operations, quicker contract negotiations, and higher levels of stakeholder trust. The emphasis on user-centric AI design is a positive development. It promotes transparency by making it easier for contract reviewers to understand and explain the AI's recommendations, ultimately fostering trust with clients and other stakeholders.
With further development of AI within these evidence-based frameworks, we might be able to uncover valuable patterns and insights into contract trends and risks. This could give organizations a head start in anticipating changes in transportation regulations and adapting their strategies accordingly. It’s a promising development, although whether it will achieve the intended level of safety and compliance remains an open question for future research.
Understanding S19A Status Requirements A Legal Analysis for AI Contract Assessment in Transportation Compliance - Real Time Compliance Monitoring Requirements for Transportation AI
The increasing use of AI in transportation is driving the need for "Real-Time Compliance Monitoring Requirements." The US Department of Transportation has established a framework to guide the use of AI in transportation, aiming to balance the benefits of AI innovation with the need for safety and responsible development. This framework emphasizes the importance of proactive risk management and ensuring AI systems consistently adhere to operational time requirements. A core element is "Operational Time Assurance" (OTA), requiring AI to monitor its own performance against safety regulations in real time. This reflects a shift towards a more automated and efficient approach to compliance, leveraging AI's ability to analyze vast datasets and provide instant alerts regarding potential violations. While these changes promise to enhance safety and efficiency in transportation, they also present challenges. Concerns around data privacy, the management of collected information, and the need for appropriate balance between encouraging innovation and adhering to regulations are critical considerations. Transportation agencies must carefully assess these issues as they integrate AI technologies into their operations.
The focus on real-time compliance monitoring in transportation AI isn't just about collecting data; it's about sophisticated algorithms capable of sifting through vast amounts of information instantly to flag potential compliance breaches as they happen. This proactive approach could theoretically lead to faster mitigation of safety hazards, which is crucial in the dynamic environment of transportation.
The demand for precise sensor accuracy highlights the need for extremely precise automated systems. Research shows that even minor deviations in automated systems can create increased risk in their predictions, reinforcing the importance of strong regulatory standards.
Regulators are increasingly pushing for real-time monitoring systems to incorporate cutting-edge machine learning. This is a recognition of the evolving landscape of transportation regulations and the need for AI systems to keep up.
While biannual audits might seem excessive, they reflect the belief that continuous oversight leads to more reliable operations. This aligns with well-established risk management principles where regular checks can help ensure robust and dependable systems.
The requirement for scenario-based training for individuals operating autonomous vehicles draws on findings from cognitive science. This research suggests that hands-on learning approaches are essential for effective decision-making in intricate situations. This is particularly critical in autonomous vehicles, where split-second decisions are often required.
The data-sharing mandates in S19A could not only speed up emergency responses but also potentially pave the way for improved interoperability among different transportation networks. This is becoming increasingly important as public transportation systems continue to integrate with each other.
Cybersecurity mandates in S19A showcase a growing trend known as "security by design." This concept is rooted in engineering best practices that suggest embedding security from the beginning of the design process, as a means to reduce risks efficiently.
The incident reporting structure is based on practices from other industries that deal with high-stakes situations. These success stories indicate that immediate data feedback systems are crucial to ensure continuous improvement in operational safety.
The restrictions on using unmanned vehicles in crowded areas likely stem from a growing body of research on human behavior and the potential for unpredictable reactions from autonomous systems in such scenarios. Without robust fail-safes, these vehicles might not respond correctly in complex, busy urban settings.
Privacy Impact Assessments (PIAs) are gradually becoming a standard practice, similar to safety checks. Research has shown that projects with well-developed PIAs tend to experience fewer compliance issues, compared to those that do not give adequate attention to data privacy early on. This signifies that proactively thinking about privacy at the outset of a project can lead to fewer problems later.
Understanding S19A Status Requirements A Legal Analysis for AI Contract Assessment in Transportation Compliance - Legal Documentation Standards for AI Contract Review Systems
The emergence of "Legal Documentation Standards for AI Contract Review Systems" signifies a crucial step toward ensuring responsible and reliable use of artificial intelligence within legal frameworks, particularly for contract review related to transportation compliance. These standards aim to establish clear protocols for the training and application of AI systems in interpreting and understanding complex legal language found within contracts. By promoting a structured approach to training AI, these standards aim to enhance the accuracy of contract review, minimize errors, and ultimately increase the efficiency of legal processes.
However, with the growing reliance on AI in legal practice, particularly in regulated industries like transportation, it's imperative to address the potential consequences of such automation. Maintaining a balance between AI-driven analysis and human oversight is crucial, especially in intricate legal contexts where nuanced interpretation and judgment remain paramount. Moreover, the implementation of these standards necessitates comprehensive documentation of AI decision-making processes and rigorous validation techniques to ensure accountability, especially when faced with compliance demands outlined in regulations such as S19A.
Successfully navigating the legal complexities of transportation law, while simultaneously mitigating potential biases and limitations in AI-driven outcomes, will depend on a robust approach that blends the strengths of both human and artificial intelligence. The ongoing evolution of AI within the legal domain will require a continuous examination of its effectiveness, ethical implications, and overall impact on legal decision-making in the context of transportation compliance.
AI contract review systems are showing promise for streamlining legal processes, particularly in industries like transportation where regulatory compliance is critical. These systems leverage machine learning to automate the scanning, interpretation, and analysis of legal documents, which can potentially free up legal teams for more complex tasks. Studies suggest AI can cut down the time spent on contract review significantly, but this gain in efficiency comes with new challenges. For instance, how do we ensure that these systems are trained in a way that avoids bias? It seems the push for evidence-based training standards aims to address this by emphasizing diverse and robust training data, hoping to minimize skewed outcomes.
The need for real-time compliance monitoring is another aspect that's gaining momentum. AI systems integrated into transportation operations could potentially react faster to compliance violations, potentially improving safety and speeding up problem resolution. However, this need for real-time insights brings its own challenges, including maintaining complete transparency in the AI's decision-making process. Under the revised S19A regulations, it seems AI-driven decisions need to be meticulously documented, allowing for easier auditing and accountability. This focus on transparency is critical in a field where errors could have significant legal ramifications.
The regulations and the constant evolution of AI systems make adaptability a critical requirement. The standards are constantly changing, and this requires the AI training protocols to keep pace with the modifications in regulations, highlighting the importance of ongoing updates and refinement. Data privacy is another significant factor, with a stronger push for anonymization techniques to protect the identities of individuals within contracts. Transportation-related AI systems must balance the need for data analysis with a responsibility to protect personal information, a critical consideration given the increasing reliance on digital platforms in legal operations.
S19A's emphasis on audits—both planned and unplanned—places a greater burden on organizations using AI systems for contract review. This increased focus on verification signifies a growing emphasis on ensuring compliance, but it also requires legal and IT teams to be well-versed in how these AI systems operate and the necessary documentation practices. However, many legal experts also suggest that human oversight remains a vital component in legal decision-making. AI's ability to interpret complex legal language and the nuances of contract context is still developing, and human judgment likely needs to play a key role in the most intricate situations.
The legal landscape is also seeing an increasing focus on cybersecurity in legal tech. As AI becomes more prevalent in contract review, the security of the underlying data becomes more crucial. This trend aligns with efforts to protect sensitive legal documents from cyberattacks, which have unfortunately become increasingly common in a digital world. As AI increasingly plays a role in contract review, questions are emerging about legal responsibility and liability if the AI makes errors or omissions. It seems the legal field is still grappling with how existing legal principles apply to AI-driven actions, requiring legal professionals to familiarize themselves with these new frameworks and implications.
While it's clear that AI offers substantial benefits in the field of contract review and legal compliance, the current push for regulation signifies a critical phase. The tension between encouraging innovation and mitigating potential risks through effective oversight remains at the forefront. As we move forward, it's likely that the roles of humans and AI in the legal profession will continue to be redefined and fine-tuned in a collaborative approach. The evolving landscape of S19A will undoubtedly continue to drive further research and development in this area.