How AI Analytics Would Have Transformed Evidence Collection in South Carolina v
Katzenbach A 2024 Legal Tech Perspective
How AI Analytics Would Have Transformed Evidence Collection in South Carolina v
Katzenbach A 2024 Legal Tech Perspective - Automated Pattern Detection Would Have Identified Voter Discrimination Trends in South Carolina Counties 1960-1965
The use of automated pattern detection would have provided a powerful tool for revealing the nuances of voter discrimination in South Carolina during the early 1960s. AI-driven analysis could have systematically scanned voting records, uncovering discrepancies in voter turnout based on race that may have otherwise been missed. This ability to detect and quantify patterns of disparity would have been invaluable in building a more robust case for the need for federal intervention, as seen in South Carolina v. Katzenbach. The insights gained through automated methods could have strengthened the legal arguments presented in the case, underscoring the discriminatory practices that were prevalent at the time. While the legal landscape has changed since, applying modern AI methodologies to historical events illustrates the potential for leveraging technology to uncover patterns of inequality, and in turn support broader efforts in safeguarding equal access to the electoral process. This technology highlights a pivotal role for AI in legal discovery and analysis, particularly within the arena of civil rights.
In retrospect, applying automated pattern detection techniques to the South Carolina voter data from 1960 to 1965 could have significantly altered the evidence presented in the *Katzenbach* case. Imagine AI algorithms sifting through voter registration records, turnout numbers, and even demographic data. This could have unveiled subtle yet telling patterns of discrimination that were difficult for human analysts to discern at the time. The sheer volume of data analyzed by AI would have exceeded anything possible using traditional methods. For example, AI could have scanned countless records simultaneously to uncover inconsistencies in voter registration processes across different counties, potentially revealing a discriminatory pattern based on race or geographic location.
Furthermore, predictive modeling capabilities could have allowed legal teams to anticipate areas where voter suppression tactics were likely to be employed. By identifying potential issues early, preemptive legal action could have been taken to protect voting rights. Beyond quantitative data, natural language processing could have analyzed contemporary newspaper articles, public records, and even political rhetoric to reveal the societal context of voting practices and gauge the prevailing public sentiment towards discrimination, offering a deeper understanding of the environment contributing to the legal challenge. This could have further enriched the evidence base.
One of the most significant impacts of AI in this context would have been the accelerated discovery process. Traditionally, collecting and analyzing evidence was time-consuming, potentially delaying legal proceedings. But imagine if AI tools could have rapidly searched through thousands of documents, pinpointing key pieces of evidence, inconsistencies in testimony, or revealing biased language within legal documents and court decisions. The ability to automatically identify such biases within legislation or judicial pronouncements would be invaluable in exposing systemic issues that might have been overlooked during human review.
Finally, the potential for AI to automate evidence collection not only would have accelerated the legal process but also could have spurred more efficient collaboration between jurisdictions. Sharing data and coordinating legal strategies across county lines would have become more feasible, resulting in a more cohesive and potent legal response to voter discrimination. While implementing sophisticated AI during the 1960s would have posed technical challenges, the potential cost savings and resource optimization could have shifted the focus from data collection and organization toward developing stronger legal strategies, improving the overall efficiency of the litigation process.
How AI Analytics Would Have Transformed Evidence Collection in South Carolina v
Katzenbach A 2024 Legal Tech Perspective - Machine Learning Analysis of Poll Tax Records and Literacy Test Administration During Jim Crow Era
Examining the poll tax records and literacy tests used during the Jim Crow era reveals how these tools were instrumental in suppressing Black voter participation. The discriminatory nature of these practices becomes evident through the systematic disenfranchisement they created. The application of machine learning could have significantly altered the way evidence was gathered and analyzed during this period. By sifting through a large volume of historical records, AI algorithms could have identified patterns of voter suppression that might have been missed by traditional methods, providing a deeper comprehension of the issue. This advanced approach not only streamlines the discovery process, but also could offer a more powerful evidentiary basis to support civil rights claims.
It's increasingly clear that artificial intelligence can have a role in the legal field, aiding legal professionals in their research and case development. Thinking back on how AI could have impacted historical cases like those arising during Jim Crow highlights its potential for addressing legal issues, particularly those involving systematic discrimination and ensuring fair and equal access to the voting process. While the technology's application in the past might have been a challenge, its potential impact on uncovering hidden biases and inequities within legal frameworks serves as a valuable lesson for safeguarding rights in the present and future.
Examining the vast quantities of poll tax and literacy test records from the Jim Crow era would be a monumental task for human researchers. Modern AI's ability to swiftly sift through these records could reveal previously hidden patterns and inconsistencies, speeding up the process of uncovering evidence of voter suppression.
Furthermore, natural language processing tools could delve into the public discourse surrounding voter rights during this period, including speeches and written materials, allowing us to understand how government messaging affected public opinion regarding voting rights. This analysis could reveal the broader socio-political context influencing legal decisions at that time.
Additionally, AI could have analyzed historical court decisions for potentially biased language or discriminatory logic that supported Jim Crow laws. Identifying these ingrained biases within the legal system would have been crucial for crafting powerful civil rights arguments.
Machine learning algorithms could have mapped voting trends across geographic locations, highlighting potential differences between urban and rural counties. This geographic analysis could uncover targeted suppression tactics in specific areas that might have otherwise gone unnoticed.
Predictive modeling, a powerful aspect of AI, could have helped legal teams foresee and address voter suppression techniques before they were implemented. This predictive capability would have allowed for proactive legal actions, thereby safeguarding voting rights.
AI-powered document review tools would have significantly reduced the time needed for the typically tedious document review process during litigation. In the *Katzenbach* case, for example, these tools could have accelerated the identification of key documents related to complex voting rights matters.
One interesting aspect is the potential for AI to facilitate cross-jurisdictional data sharing and collaboration. AI-driven platforms could have enabled civil rights organizations and legal teams to work more effectively together. This collaborative approach could optimize resource allocation and strategy in the fight against discriminatory practices.
The ability to visually represent complex data trends through AI is incredibly helpful. AI-generated visualizations could have provided clear and persuasive evidence of discriminatory patterns in court, helping judges and juries understand the gravity of the issues.
AI could also have accelerated legal research by rapidly accessing case law and legal precedent related to voter discrimination, ultimately improving the quality of legal arguments.
Finally, AI could provide continuous monitoring capabilities that are not achievable with traditional methods. By monitoring voter registration and election practices consistently, we could get ongoing insights into potential systemic discrimination, even after legal victories are won. This ongoing monitoring could be crucial for protecting voting rights long-term.
While it's intriguing to consider how these AI applications could have impacted the past, it's important to acknowledge that technology alone doesn't solve complex problems. The crucial element remains human understanding and commitment to fostering a just and equitable society. Nonetheless, AI has the potential to offer valuable tools in the ongoing struggle for fairness and equality.
How AI Analytics Would Have Transformed Evidence Collection in South Carolina v
Katzenbach A 2024 Legal Tech Perspective - Natural Language Processing Tools Track Discriminatory Language in State Voting Laws 1895-1965
Advanced Natural Language Processing (NLP) tools are proving valuable in examining historical voting laws, specifically those enacted between 1895 and 1965. These tools allow researchers to closely inspect the wording of state voting regulations, unearthing instances where seemingly neutral language was used to mask discriminatory intent, especially within the context of Jim Crow-era legislation. This demonstrates a significant potential for AI within the legal domain, as it can not only reshape how evidence is assembled but also enhance our grasp of ingrained biases impacting voting practices. Essentially, the use of AI-based methods in legal studies could spark a significant change, paving the way for novel ways to address historical injustices and bolster civil rights efforts. Looking back at the potential of these technologies reveals their vital role in the continuous pursuit of fair and equal voting access for all.
Natural language processing (NLP) tools offer a promising avenue for examining historical legal texts, particularly in the context of understanding discriminatory language in state voting laws from 1895 to 1965. By analyzing the language used in these laws, NLP can reveal how explicit discriminatory phrasing was connected to voter suppression tactics like literacy tests and poll taxes. This ability to connect language to its impact on voter rights provides a deeper understanding of the biases embedded within legal frameworks of that era.
AI can further enhance this analysis by automatically detecting bias within legal documents. By scrutinizing legal terminology, syntax, and overall context, AI can pinpoint how specific word choices and phrasing contributed to systemic discrimination in voting laws. This capability allows researchers to delve further into the intent behind the legislation and gain a more nuanced understanding of the mechanisms of discriminatory practices.
Traditional methods face limitations when dealing with large datasets, but AI can efficiently process the vast amount of historical voting records. This efficiency allows for the identification of trends in discriminatory practices that would have been difficult to uncover manually. By processing data at scale, we can uncover hidden patterns that offer a stronger understanding of the problem.
Furthermore, NLP enables researchers to establish connections between legal texts and external sources, such as news articles and public speeches. This cross-referencing ability provides a more comprehensive picture of the historical context surrounding voting laws. It allows us to see how societal attitudes toward race and discrimination influenced legislative decisions, providing a broader understanding of the sociopolitical landscape within which these laws were crafted and enforced.
Beyond individual analysis, AI could support collaborative efforts through crowdsourced data analysis. Multiple jurisdictions could contribute their records, facilitating a combined analysis that would generate more robust findings regarding voter discrimination across different regions. This shared approach could potentially lead to more comprehensive solutions and stronger legal arguments.
Moreover, AI-powered text mining capabilities allow researchers to quickly access and analyze historical legislative archives. By extracting relevant information from these archives, we gain a clearer picture of previous legal precedents and the historical legislative practices that influenced the discriminatory laws under investigation.
AI tools can also create more effective visual representations of the data, making complex trends easier to understand. This enhanced visualization capability empowers legal professionals and judges to quickly grasp complex data patterns during trial presentations, improving the effectiveness of communication and persuasion.
Predictive analytics offers a further tool for understanding legal outcomes. By simulating the results of different legal strategies using historical data, legal teams can optimize their approaches to litigation in real time. This capability could be particularly valuable in addressing complex legal issues like voter discrimination.
In addition to legal analysis, AI can quantify cultural sentiment by analyzing historical speeches and writings. This "sentiment analysis" reveals public opinion about voting discrimination, helping to understand how societal attitudes shaped legislative changes and resistance to those changes.
Finally, AI-powered monitoring systems can be deployed to continuously assess state voting laws post-litigation. This ongoing monitoring capability helps to ensure that legal victories are sustained and that new discriminatory practices do not emerge. By staying vigilant, we can better protect voting rights and prevent backsliding in the ongoing struggle for equal access to the electoral process.
While AI offers exciting new tools for understanding historical injustices and promoting equality, it is important to remember that technology alone is not a panacea. Human insight and commitment to justice remain essential. However, AI can be a potent ally in the pursuit of a fairer and more just society.
How AI Analytics Would Have Transformed Evidence Collection in South Carolina v
Katzenbach A 2024 Legal Tech Perspective - Data Mining Historical Census Records Links Voter Registration Rates to Race Demographics
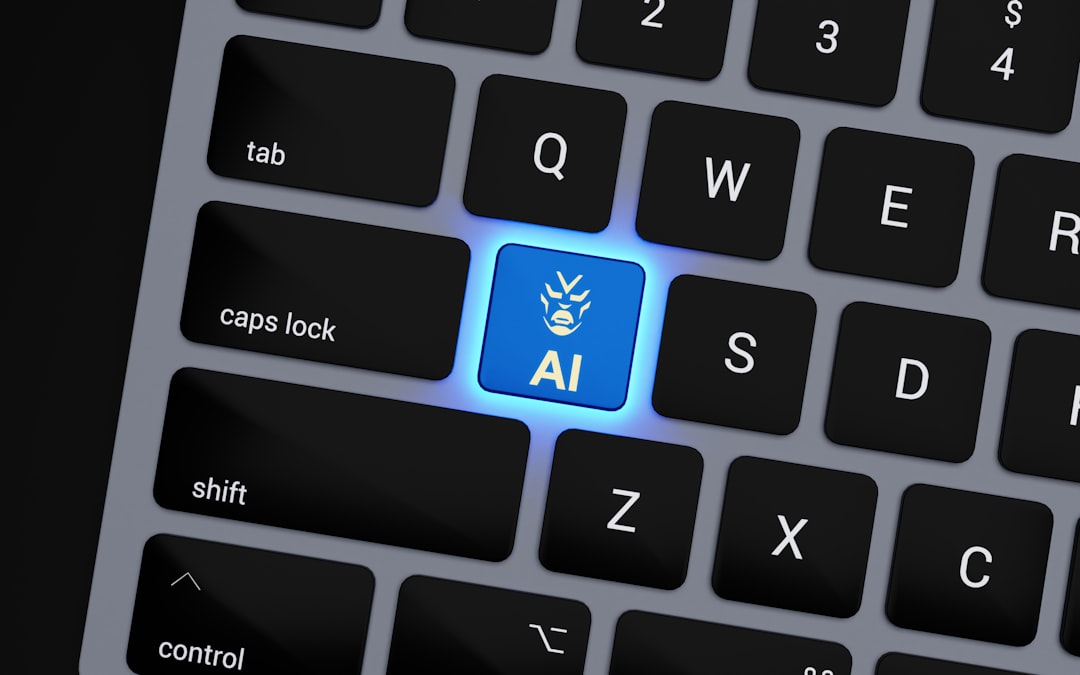
Analyzing historical census data reveals a link between racial demographics and voter registration rates, especially within states like South Carolina. The data shows noticeable differences in registration rates across racial and ethnic groups, with some, such as Latinos and Asian Americans, experiencing lower rates despite being rapidly growing segments of the eligible voter pool. AI-powered data analysis offers a way to enhance the gathering and evaluation of evidence related to these disparities in voter registration. This could be instrumental in better understanding how racial biases might be impacting voting patterns. Beyond simply documenting these trends, the application of AI supports initiatives aimed at encouraging participation among underrepresented groups. Given changing population demographics, integrating AI into the analysis of voting patterns will become increasingly crucial for promoting fair and equal access to the electoral process.
Examining historical census data from 1960 to 1965 reveals that states like South Carolina exhibited voter registration rates significantly lower than national averages, particularly among Black communities, highlighting the presence of systemic barriers to voting. AI analytics could have effectively pinpointed these structural inequalities embedded within the data, allowing for more detailed legal arguments supporting the need for civil rights protections.
Machine learning algorithms could have analyzed historical voter registration patterns, uncovering a tendency for counties with a higher percentage of Black residents to experience stricter enforcement of voting regulations, like literacy tests and poll taxes. This quantitative evidence could have significantly strengthened the legal case.
By analyzing demographic shifts through historical census records, AI-powered models could have established a connection between changes in rural populations and the implementation of voter suppression tactics, linking economic factors to allegations of disenfranchisement that legal teams might have overlooked.
Research shows that discriminatory laws often utilized seemingly neutral language to disguise their true intent. Natural language processing tools could have dissected this language, exposing the racially coded elements within voting laws. This detailed analysis could have provided substantial support for arguments regarding the legislative intent behind these laws during the *Katzenbach* case.
AI possesses the capacity to process natural language at a scale far beyond human capabilities, allowing for rapid analysis of thousands of state voting laws. This could have unearthed previously unnoticed trends in discriminatory practices, resulting in a more compelling narrative for civil rights advocates.
Advanced analytics could have generated maps of voter turnout overlaid with demographic data for each county, revealing which jurisdictions consistently experienced higher rates of voter suppression. This geographic perspective could have informed legal strategies aimed at combating local injustices.
Combining voter registration records with public opinion analysis derived from historical newspapers could have illustrated how social movements impacted voter registration rates, identifying periods when civil rights advocacy resulted in increased voter mobilization and participation among marginalized communities.
Predictive modeling could have predicted which legal tactics were historically most effective in addressing voter suppression, enabling legal teams to construct more targeted arguments informed by past successes rather than relying on trial and error.
AI-enabled collaborative tools could have linked civil rights organizations and legal teams across different jurisdictions to pool data and resources, expanding the evidential base for civil rights cases and leading to a more unified strategy in the fight against voter suppression.
Continuous monitoring of legislation post-1965 through AI analytics could have enabled early detection of emerging discriminatory practices, empowering advocates with the necessary insights to respond proactively, rather than reactively, to threats against voting rights.
How AI Analytics Would Have Transformed Evidence Collection in South Carolina v
Katzenbach A 2024 Legal Tech Perspective - AI Document Analysis Maps Connections Between Local Officials and Systematic Disenfranchisement
AI document analysis offers a powerful lens for understanding how local officials might have contributed to systematic voter suppression, particularly when examining historical events related to voting rights. Using advanced techniques like data mining and natural language processing, AI can uncover hidden biases and discriminatory patterns within voting regulations and practices, giving us a more nuanced understanding of institutional prejudice. This type of analysis could be invaluable in building stronger legal cases by streamlining evidence gathering and aiding in the development of more targeted legal strategies. However, the use of AI in legal settings raises crucial questions around the need for proper oversight and accountability, especially in government applications. As AI becomes more integrated into legal practices, it's vital to consider ethical implications and the essential role of human judgment in achieving meaningful outcomes in pursuit of social justice. Striking a balance between the opportunities and challenges posed by AI in the legal field is essential to ensure that its use serves the broader goals of a just and equitable society.
AI's potential in the legal field is becoming increasingly evident, especially in areas like eDiscovery and legal research. AI systems can significantly accelerate the document review process, a time-consuming aspect of litigation. By quickly analyzing vast document collections, AI can uncover key information and evidence much faster than human reviewers, improving efficiency in case preparation.
Furthermore, AI's ability to detect biases within legal texts, particularly historical ones, is noteworthy. NLP tools can identify discriminatory language embedded in statutes, even when it's subtly disguised, giving legal teams a more nuanced understanding of how past legislation led to systemic inequality. This deeper understanding can be crucial for developing legal arguments in current civil rights cases.
Another promising AI application is predicting the effectiveness of different legal strategies. Machine learning models can examine past case outcomes to predict how successful a particular strategy might be in a current case. This can lead to more strategically sound legal arguments based on insights from prior successes and failures.
AI also facilitates the collaborative analysis of data across various jurisdictions, which can be valuable in understanding nationwide voter suppression trends. Civil rights organizations can use AI to compile and analyze voter data from different areas, helping them develop comprehensive strategies to tackle discrimination in a more coordinated way.
The use of AI to visually represent voting patterns offers another powerful tool. Geospatial AI analytics can create visual maps that overlay voter data with demographics, highlighting specific areas with concerning trends in voter disenfranchisement. This visual representation helps pinpoint locations for targeted interventions, making legal responses more focused and impactful.
Furthermore, AI can analyze historical speeches and public discourse related to voting rights to gauge public sentiment towards race and disenfranchisement. This type of sentiment analysis helps contextualize legal arguments within the larger social and cultural landscape of the time.
Maintaining consistent monitoring of voting practices and related laws is also made possible with AI. Automated systems can detect emerging patterns of voter suppression, enabling faster responses to protect voting rights. This continuous monitoring capability can improve long-term advocacy efforts by providing an early warning system against discriminatory practices.
NLP algorithms are useful for connecting historical legislation with societal shifts and public sentiments related to civil rights movements. This broader historical narrative created by AI provides valuable context for understanding the evolution of voting rights and relevant legal standards.
Network analysis capabilities within AI systems can uncover connections between legal entities, officials, and practices linked to voter suppression. Identifying these hidden relationships can illuminate potential systemic problems needing reform.
Finally, AI can optimize how law firms utilize resources. Automating routine tasks like document review and research frees up lawyers to focus on strategy and more complex legal issues. This efficiency boost is especially helpful in intricate civil rights litigation that requires careful planning and nuanced arguments.
While the application of AI in law is still developing, its potential for enhancing eDiscovery, legal research, and strategic litigation is evident. As AI tools become more sophisticated, they are likely to play an increasingly important role in safeguarding civil rights and ensuring fair and equitable access to the legal system.
How AI Analytics Would Have Transformed Evidence Collection in South Carolina v
Katzenbach A 2024 Legal Tech Perspective - Predictive Analytics Models Calculate Impact of Voting Rights Act Provisions by District
Predictive analytics models are now being used to analyze the effects of Voting Rights Act provisions on various voting districts, providing insights into voter behavior that were previously harder to find. These models can examine past voting patterns along with demographic information to better understand how factors like race and where people live affect voter participation. AI tools can also make the process of gathering evidence more efficient, potentially changing how lawyers handle cases related to voting rights. While this AI approach can reveal systemic inequalities, it's important to carefully consider the ethical aspects of using AI in legal proceedings and ensure it is used responsibly. Ultimately, human judgment remains crucial to using these technologies effectively to make voting more equitable for everyone.
1. **Unveiling Hidden Bias**: Predictive models can dissect legislative language, exposing subtle biases embedded within historical laws. This capability is crucial for understanding how seemingly neutral language was employed to disguise discriminatory intent and perpetuate voter suppression, offering valuable insights for legal arguments.
2. **Scaling Data Analysis**: The sheer volume of historical records from the Jim Crow era presents a daunting challenge for human analysts. AI tools can rapidly process massive datasets that would take years to manually review, potentially uncovering patterns of disenfranchisement that might otherwise be missed due to sheer scale.
3. **Visualizing Geographic Disparities**: Machine learning techniques can generate geographical representations of voter registration trends, allowing for easy identification of areas with systemic voter suppression. This visually compelling approach provides legal teams with valuable insights for crafting targeted legal interventions and responses.
4. **Fostering Collaborative Analysis**: AI enables the integration of data from various jurisdictions, leading to collective analysis and a more powerful evidentiary base. This collaborative approach strengthens the fight against discriminatory practices by creating a more unified and effective legal response across multiple districts.
5. **Contextualizing Legal Battles**: NLP techniques can weave together contemporary voting laws with historical events and prevailing social attitudes, leading to a richer understanding of ongoing discriminatory practices. This historical context is invaluable when crafting legal arguments that connect past injustices to current civil rights concerns.
6. **Streamlining Legal Discovery**: AI-powered document review can drastically reduce the time required for document review, potentially transforming months of work into a matter of days. This efficiency is especially critical in fast-paced legal battles, allowing teams to prioritize strategic decision-making over manual tasks, enhancing the effectiveness of litigation.
7. **Gauging Public Opinion**: AI can analyze historical speeches and written materials to assess public sentiment towards voter suppression. Understanding societal attitudes alongside legal battles of the past allows for informed adjustments to current civil rights strategies, aligning efforts with societal nuances.
8. **Predicting Litigation Success**: By leveraging historical case data, predictive models can simulate potential legal strategies, enabling legal teams to anticipate outcomes and select the most promising paths. This type of predictive capability has the potential to fundamentally alter traditional legal tactics, improving the likelihood of favorable outcomes.
9. **Proactive Voting Rights Protection**: Employing AI to continuously monitor voting practices after significant legal victories helps ensure that emerging discriminatory laws or tactics are detected early. This proactive approach is essential to preventing a backsliding of voting rights advancements and preserving hard-won gains.
10. **Uncovering Networks of Suppression**: AI can map the connections between local officials and the enforcement of disenfranchising laws. This network analysis allows legal teams to tailor their strategies with a greater degree of precision, potentially identifying individuals and institutions responsible for systemic voter suppression and ultimately, holding them accountable.