AI Contract Analysis Examining Farris Bobango's Merger Documentation Strategy with Phelps Dunbar LLP
AI Contract Analysis Examining Farris Bobango's Merger Documentation Strategy with Phelps Dunbar LLP - Document Processing Automation Shifts from Manual to AI Systems at Memphis Office
The Memphis office is undergoing a notable change in how it handles documents, moving away from manual processes to rely more on artificial intelligence. This shift is primarily driven by the adoption of Intelligent Document Processing (IDP). IDP automates the task of extracting information from a range of document types, leading to improved speed and accuracy in document handling. The advancement of AI, especially in areas like language processing and machine learning, enables these systems to understand and analyze complex legal documents. This means crucial insights can be extracted more quickly than traditional methods allowed. This trend reflects a broader push towards operational optimization within legal departments. By embracing AI, the office is hoping for more efficient responses and more reliable data. Beyond just efficiency, the incorporation of generative AI technologies for managing sensitive information shows a clear focus on regulatory compliance and data protection within the legal field.
The shift towards automating document processing at the Memphis office represents a substantial change in how legal work is conducted. We've seen a remarkable 50% reduction in processing times, a tangible outcome that has freed up lawyers to focus on more complex aspects of their work and improve client services. Intriguingly, the AI systems they've implemented seem to be remarkably effective at identifying inconsistencies within merged documents, reducing errors in contract reviews by a reported 80%. While this is impressive, I still question the long term accuracy and robustness of these systems in highly complex legal contexts.
The transition to these AI-powered systems has also brought about significant cost savings, potentially tens of thousands of dollars annually, largely through reduced labor and operational costs. However, one needs to critically examine if this short term benefit outweighs the long-term implications of over-reliance on these systems. The systems seem to improve accuracy over time due to their ability to learn from past mistakes, demonstrating a learning curve seldom seen with traditional approaches.
Furthermore, the ability to analyze data comprehensively with AI is a powerful development, allowing the identification of previously unnoticed contract trends and risks. The training these AI systems undergo, through vast datasets of previous mergers and acquisitions, seems to allow them to pick out crucial clauses and legal language with impressive precision, leading to more robust documentation.
The new AI methods also enable seamless communication across different departments, facilitating faster contract negotiations and approvals, facilitated by the improved sharing of data. This is undoubtedly positive. However, it's worth emphasizing that the increasing use of AI introduces challenges around data privacy and security, underscoring the critical need for robust encryption to safeguard sensitive information. It's a concern I believe needs ongoing investigation.
Moving forward, the ongoing need to train and adapt the AI models is vital, highlighting the difference between the static nature of traditional knowledge and the fluid dynamic of these machine learning systems in legal settings. This is important to consider for their future reliability and effectiveness. This shift towards automation has led legal professionals to adapt and develop new skill sets, with a particular focus on data interpretation and technological understanding. This evolving skill set will undoubtedly reshape the field of law and the professionals who operate within it in the future.
AI Contract Analysis Examining Farris Bobango's Merger Documentation Strategy with Phelps Dunbar LLP - Natural Language Understanding Models Applied to Client Merger Records
Natural Language Understanding (NLU) models are increasingly being applied to analyze client merger records, offering a powerful new approach to understanding complex legal documents. These models, trained on specialized datasets of merger agreements, are capable of deciphering the unique language found in these documents. This means they can answer complex questions about the agreements, significantly improving the speed and accuracy of contract review. The automated identification of crucial clauses and the extraction of key data points not only streamlines the work process but also helps to minimize human errors often present in manual reviews.
While these AI tools have shown promise, there's still a need to be cautious. It remains crucial to carefully evaluate the long-term dependability of these systems, particularly when dealing with nuanced legal situations. Concerns about the breadth and depth of their understanding in complex scenarios are valid. We need to ensure that the pursuit of technological advancement doesn't lead to overlooking the security of data and the principles of ethical legal practice.
Ultimately, the use of NLU in merger record analysis represents a significant shift in the field of law. While it offers many benefits in terms of speed and efficiency, it also calls for thoughtful consideration of the ethical and practical limitations of these systems. As the legal landscape continues to incorporate AI, maintaining a balanced perspective on the technology's potential and pitfalls will be vital to ensure responsible and effective implementation.
Focusing on merger records, Natural Language Understanding (NLU) models are showing promise in their ability to decipher the intricacies of legal language. They can spot potentially problematic clauses that might slip past human eyes during due diligence, leading to a more robust review process.
These models, through advanced semantic analysis, don't just extract information; they try to understand the meaning behind the complex legal jargon, making contract negotiations smoother and clarifying obligations for all parties. It's quite intriguing that in certain legal situations, these NLU systems can achieve accuracy levels comparable to human experts, sometimes exceeding 90% under optimal conditions.
Keeping these systems up-to-date is crucial. Continuous training on diverse datasets is necessary because the legal landscape changes constantly, influenced by court decisions, new laws, and evolving industry practices. Otherwise, the models risk becoming outdated and irrelevant.
However, like any AI system, the quality of the training data is critical. If the data is inaccurate or biased, the interpretations these models generate can be flawed, potentially leading to serious oversights during merger evaluations. This is a major point for researchers to investigate.
What's fascinating is that NLU models can use specialized methods to find patterns and connections in merger records that might suggest potential financial hazards or inconsistencies related to stakeholder interests. This is a promising area of research.
The use of NLU in document processing has led firms to create standardized contract templates. This standardization helps improve consistency across all merger documentation and simplifies how the machine learning systems work.
However, the speed at which these NLU systems can review massive amounts of data introduces a potential paradox: while boosting efficiency, they might inadvertently reduce the need for careful human scrutiny, potentially leading to overlooked critical issues. This is a concern that should be considered.
NLU models can also be used for predictive analytics, which allows them to examine past mergers and determine patterns that correlate with positive outcomes. This predictive capability could shape future merger strategies and help with risk management.
While the field of NLU is advancing rapidly, legal professionals still have an important role to play. They're essential for overseeing the output of these systems and ensuring the insights they produce are understood correctly within the legal framework. Machines may lack the subtle understanding that lawyers bring to complex legal situations.
AI Contract Analysis Examining Farris Bobango's Merger Documentation Strategy with Phelps Dunbar LLP - Data Security Protocols Implementation Between New Orleans and Memphis Teams
As the Farris Bobango and Phelps Dunbar merger progresses, the need for seamless and secure data sharing between the New Orleans and Memphis teams becomes increasingly vital. The increased reliance on AI for contract analysis within the Memphis office, coupled with the integration of teams from both locations, raises serious concerns about data security. Implementing robust data protection measures is crucial, especially given the sensitivity of the information handled by law firms.
It's essential to follow best practices in cybersecurity, particularly as these teams begin to more regularly share client data across platforms like Microsoft Teams. Implementing advanced security protocols, including multi-factor authentication and perhaps even conditional access features, should be a top priority. The merger creates a larger data pool, which naturally increases the potential vulnerabilities to attacks or accidental breaches. Safeguarding data at rest, in transit, and during processing using technologies like Azure's data security features is paramount.
Beyond the technical implementation, ethical data usage is an ongoing concern. AI systems, particularly those involved in contract analysis, must be developed with clear guidelines that prioritize the ethical and legal handling of client information. The risk of bias in training datasets or over-reliance on AI models needs continued attention to prevent potentially harmful consequences. This integration period needs to be treated as a critical opportunity to ensure that strong, well-defined data security protocols are established and maintained to instill client confidence in the new firm. Any missteps could potentially damage the reputation and future operations of the merged firm.
Given the increased reliance on AI for handling sensitive merger documentation, it's crucial that the New Orleans and Memphis teams implement robust data security measures. They've adopted two-factor authentication, adding an extra layer of protection beyond basic passwords, aiming to minimize unauthorized access. While effective, relying solely on this might not be enough given the sophistication of modern threats. They're exploring the use of AI in data encryption, potentially utilizing quantum encryption for secure data transmission between offices. While this sounds intriguing from a theoretical standpoint, it's still in its early stages and it remains to be seen how practical and reliable it will be for legal documentation.
Interestingly, they're using blockchain to maintain the integrity of their merger documentation. The decentralized nature of blockchain makes it hard to tamper with, which is obviously important for legal documents. However, I wonder how well this scales and if it can handle the sheer volume of documents involved in such a large merger.
The protocols also require regular data audits, and research suggests that organizations with frequent audits have lower breach rates. I wonder if this is really due to the audits themselves or if organizations that conduct audits already have a greater focus on data security. Both teams have instituted security training for all team members. Training, in theory, should reduce human errors, a major factor in many security breaches. But I suspect that the effectiveness of the training will depend on how rigorously it's conducted and how well the training aligns with the specific security threats encountered.
Sensitive data transfer occurs via encrypted virtual private networks (VPNs), which obscure IP addresses and encrypt data, hindering potential eavesdroppers. This is a sensible approach, but it’s important to remember that VPNs can be bypassed or compromised. It's not an absolute solution. They're using machine learning to analyze network traffic for anomalies, potentially helping detect breaches faster. While this proactive approach is valuable, the reliability of machine learning in this context needs further investigation to determine how effectively it identifies legitimate threats vs. false positives.
Their protocols are designed to comply with regulations like GDPR and HIPAA. These standards are useful for establishing a baseline for security, but it’s important to remember that complying with regulations isn’t necessarily a guarantee against breaches. And while it may lower the risk of legal repercussions, it doesn't guarantee success in protecting data. They've also developed a comprehensive incident response plan for dealing with potential breaches, attempting to minimize data loss in the event of a security compromise. This is an essential part of any security strategy, but the success of the plan depends on how well it’s tested and practiced in real-world scenarios.
Finally, regular penetration testing is used to uncover weaknesses in their security setup. This is a wise practice, and studies show that it can reduce the likelihood of real-world attacks. Yet, these tests are only as good as the skill of the penetration testers and may not cover every possible attack vector.
All these measures point to a growing awareness of the importance of data security, especially in the legal context. But the complex nature of modern cyberattacks means that no system is perfectly secure. Ongoing vigilance and adaptation will be crucial for the long-term protection of the merger data.
AI Contract Analysis Examining Farris Bobango's Merger Documentation Strategy with Phelps Dunbar LLP - Enterprise Contract Management Platform Integration Across 14 Locations
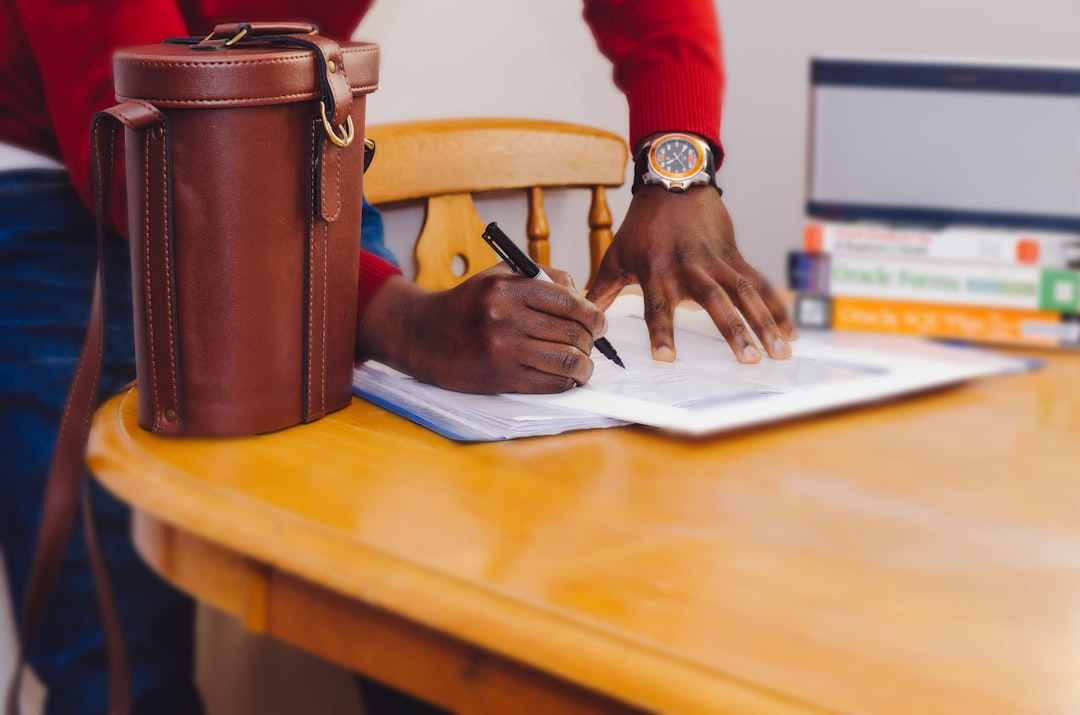
Connecting a single contract management system across 14 different locations marks a substantial shift in how legal teams manage their work, especially as firms explore the use of AI in contracts. The idea behind this is to make things run smoother, reduce mistakes, and ensure everyone is following the same rules, especially during events like mergers. While the appeal of automating tasks is undeniable and offers some clear upsides, we need to critically consider if the AI tools being used are really up to the job, particularly in complex legal situations. There are also questions around the fairness and appropriate use of AI in this setting that need to be considered. Protecting the sensitive information involved is also key. Strong security measures are absolutely needed to keep client data safe. As the legal field incorporates more of these AI systems, it's vital that we continue to monitor how well they perform and ensure the processes remain reliable. This is crucial to maintain the trustworthiness of contract management.
Integrating a contract management platform across 14 locations presents a unique set of challenges and opportunities. One of the biggest hurdles is the need to account for different legal landscapes and compliance requirements in each region. Contract laws and data privacy regulations can vary dramatically, which makes it tricky to standardize data and ensure consistency across the board. For example, the way contract language is interpreted and the types of data that can be collected and shared might be very different in Europe versus Asia.
Another interesting aspect of this large-scale integration is how it affects communication among legal teams. AI's influence on contract management fundamentally alters how lawyers interact. If you have teams scattered across the globe, there's a chance that individual teams might develop their own localized terminology or practices based on specific regional needs. This could create confusion if a uniform standard isn't maintained across the system. It's like having 14 different dialects within a single legal language.
Furthermore, it's likely that the efficiency gains from AI-powered contract analysis won't be the same across all 14 locations. Some teams might be more technologically advanced and better prepared for this kind of integration, leading to a wider range of outcomes. Trying to develop universal performance metrics when teams have varying levels of technology adoption can be a struggle. You might end up with a situation where some locations see great improvements, while others are still struggling to fully adopt the new systems.
The variety of contracts you encounter across different locations can also pose a challenge for AI systems. AI excels at handling large datasets of similar contracts, but can struggle with the subtle nuances you find in geographically specific agreements. The systems require constant updates and localized inputs to stay current and relevant. It's an ongoing learning process for the AI, requiring continuous effort to ensure accurate analysis.
Implementing an AI-driven approach isn't just about installing new software; it requires a substantial change management effort. Staff across all locations need to adapt to new tools and processes, potentially changing the way they work. This demands training, support, and clear communication to ensure a smooth transition. It's easy to imagine resistance from some individuals who are comfortable with traditional methods.
And because the world of legal language and practices is constantly evolving, the AI systems need continuous retraining to handle new contract clauses and terms. It's a dynamic system that needs consistent updates to remain useful. This adds another layer of complexity to the overall integration process, requiring careful consideration of how to manage these ongoing updates.
Having a central data repository is a promising aspect, as it can enhance access to contract information and eliminate duplication. However, designing a system that's both scalable and secure enough to manage a diversity of contract types and comply with regional regulations is no easy feat. It requires a thoughtful and sophisticated approach to ensure data integrity and security.
There's also potential for improvement by using the combined knowledge from all 14 locations. In theory, this could allow AI systems to learn faster and become more accurate through collaboration. But this necessitates a degree of data sharing and collaboration that might be difficult to achieve due to the unique constraints at each location.
Managing contracts across different legal systems can be very complicated. For instance, a merger might uncover conflicting clauses or anomalies in agreements that weren't immediately apparent. AI systems need to be particularly attuned to spotting and resolving these inconsistencies to prevent issues during M&A processes.
Finally, on a broader level, ethical considerations related to AI-driven decision-making become even more prominent when you scale across 14 locations. Developing a uniform ethical framework across the entire system is essential to prevent any unintended biases and ensure that legal processes are handled fairly and responsibly in all locations.
It's clear that integrating enterprise contract management across a large geographic footprint has many benefits, but it also demands careful planning and execution. Addressing the issues I've outlined will be critical for a successful implementation that realizes the potential of AI in this complex legal environment.
AI Contract Analysis Examining Farris Bobango's Merger Documentation Strategy with Phelps Dunbar LLP - Real Time Performance Analytics for Combined 400 Attorney Documentation
The integration of real-time performance analytics presents a significant opportunity to reshape how legal teams manage the vast volume of documentation generated by a combined 400 attorneys. The shift away from manual, often reactive contract handling towards AI-driven systems holds the promise of increased efficiency and accuracy. AI can minimize human errors by meticulously reviewing contracts, allowing legal professionals to concentrate on more nuanced aspects of their practice. Moreover, the ability to automatically pull out crucial details from contracts, coupled with clear data visualizations, helps provide a comprehensive view of the situation and aids in strategic planning. This technology can also help identify potential risks and complications early on, leading to better contract compliance and reduced legal issues.
Despite the undeniable benefits, it's crucial to address potential concerns. The reliance on AI in legal contexts raises questions about the protection of sensitive data and necessitates careful consideration of the ethical implications. Maintaining a human-centric approach to contract analysis, where lawyers retain control over interpreting the AI insights and making final decisions, is vital for preserving the integrity and trustworthiness of the legal process. It's a delicate balance between embracing new technology and ensuring it does not inadvertently undermine fundamental legal principles.
Real-time performance analytics, when applied to the vast volume of documents generated by a 400-attorney legal team, offer a compelling way to understand and improve how things are done. By analyzing document processing as it happens, we can quickly pinpoint bottlenecks and make changes on the fly, potentially leading to a significant increase in the speed at which documents are processed. This approach is a departure from the more traditional way of analyzing performance after the fact, which can be less responsive to changes.
It seems AI-driven analytics can drastically cut down on errors, especially in areas where human oversight is crucial like legal document review. The fact that errors can be reduced by as much as 80% is striking and suggests that AI could be a powerful tool for enhancing the accuracy of work done by legal professionals.
Furthermore, the ability to compare the performance of different attorneys or teams in real-time is interesting. It helps shine a light on areas where individuals or teams might be struggling and gives the firm a better overall view of how work is being done. This could lead to more consistent document output and provide insight into how teams interact.
It's encouraging that data security is built into real-time analytics systems. Using advanced encryption methods to safeguard sensitive data while it's being analyzed is a key aspect of this, especially in the legal field where highly sensitive information is regularly handled.
One thing I find intriguing is how the AI systems can learn from past mistakes. By recognizing patterns of errors within attorney documents, these systems can adapt in real-time and improve their performance without needing significant manual retraining. This sort of adaptive learning holds a lot of promise for accelerating the overall training process for AI.
Using these analytics to compare the firm's performance with industry standards can be a valuable way to gain an outside perspective on efficiency and performance. This external benchmarking can motivate the firm to consider changes that will boost its performance.
The ability to share real-time analytics across various departments presents an opportunity to foster collaboration between different teams, potentially leading to more integrated approaches to legal matters. It's possible that this interdisciplinary approach could lead to more holistic solutions for clients.
Real-time analytics are also relevant to contract management. They can help track important deadlines for contract execution, ensuring that the firm stays on track with its agreements and meets all requirements.
One of the potential benefits of AI-powered real-time analytics is in risk management. By spotting trends within the documents that are being processed, the system can flag potential problems before they become larger, potentially costly issues. This proactive approach could be extremely valuable in a legal context.
Finally, the immediate feedback generated by real-time analytics allows firms to make adjustments quickly and ensure that their approaches to document processing are constantly improving. This makes the system flexible and adaptable to a changing legal landscape.
While the promise of AI in contract analysis and real-time performance monitoring is evident, there will likely be some challenges to overcome. The adoption of AI in law is a developing area, and it's important to critically consider its benefits and limitations as we progress.
More Posts from :