7 Critical Ways AI is Transforming Digital Evidence Authentication in Frame-up Cases
7 Critical Ways AI is Transforming Digital Evidence Authentication in Frame-up Cases - Blockchain Integration Authenticates Digital Evidence Chain of Custody Through 2024
The increasing reliance on digital evidence in legal proceedings necessitates robust methods for maintaining its integrity and authenticity. Blockchain technology, with its immutable record-keeping and transparency, offers a powerful solution for managing the chain of custody for digital evidence. Approaches like LEChain exemplify this by leveraging blockchain's inherent features to enhance control over evidence handling, ensuring its authenticity and traceability. Moreover, the introduction of encrypted access controls through permissioned blockchain networks adds another layer of security, protecting sensitive evidence from unauthorized access. While the potential benefits of blockchain are evident, the integration of AI further augments its effectiveness. AI-driven tools can enhance the analysis and interpretation of digital evidence, helping identify patterns and anomalies that might otherwise be missed. This synergy is particularly valuable in complex cases, such as those involving potential frame-ups, where a meticulously documented and transparent chain of custody is crucial to establishing the reliability of the evidence. The combined potential of AI and blockchain technology offers a promising outlook for evidence authentication in legal settings. As we approach 2024, expect to see further advancements in these areas, leading to a more reliable and efficient system for managing digital evidence.
Existing database systems are often inadequate for guaranteeing the integrity and authenticity of digital evidence, which has spurred the exploration of blockchain solutions. A potential approach involves utilizing a ciphertext-policy attribute-based encryption protocol for authenticating and safeguarding digital evidence while preserving its confidentiality.
However, current implementations predominantly rely on storing metadata about the evidence on the blockchain, while the evidence itself resides on separate platforms, only accessible to designated individuals. This setup raises questions about the practicality of blockchain's purported immutability in certain scenarios.
The promise of blockchain lies in its potential to standardize the chain of custody process in digital forensics, a pivotal aspect in securing successful legal outcomes. Specifically, a blockchain-based approach was recently designed for handling the chain of custody related to fitness trackers in digital forensic investigations, aiming to promote both data integrity and transparency.
The LEChain framework is a testament to the possibilities of using blockchain to improve control over the entire chain of custody of digital evidence. This includes prioritizing the attributes of transparency, immutability, and verifiability. LEChain leverages short randomizable signatures to anonymously validate the identities of witnesses, further securing the process.
Restricting access to a blockchain network through a permissioned and private design is a common strategy to protect the integrity of the system and evidence. This is crucial in the context of digital forensics and legal proceedings.
It's noteworthy that AI is being increasingly incorporated into the authentication of evidence, especially in situations where evidence manipulation is suspected. This enhanced AI involvement holds great potential to improve the reliability and accuracy of evidence handling. The rise in criminal activities that involve digital evidence necessitates a robust and verifiable system, and blockchain is viewed as a compelling solution for ensuring investigative integrity.
While blockchain's potential for improving legal practices is intriguing, it's still relatively early in its adoption. The legal landscape is just beginning to explore and consider its implications, potentially creating a need for new regulations and procedures surrounding the use of AI and blockchain technologies. How these technologies will ultimately impact legal proceedings and the integrity of evidence is an ongoing area of inquiry and exploration.
7 Critical Ways AI is Transforming Digital Evidence Authentication in Frame-up Cases - Machine Learning Algorithms Detect Manipulated Video Evidence in State v Miller Case
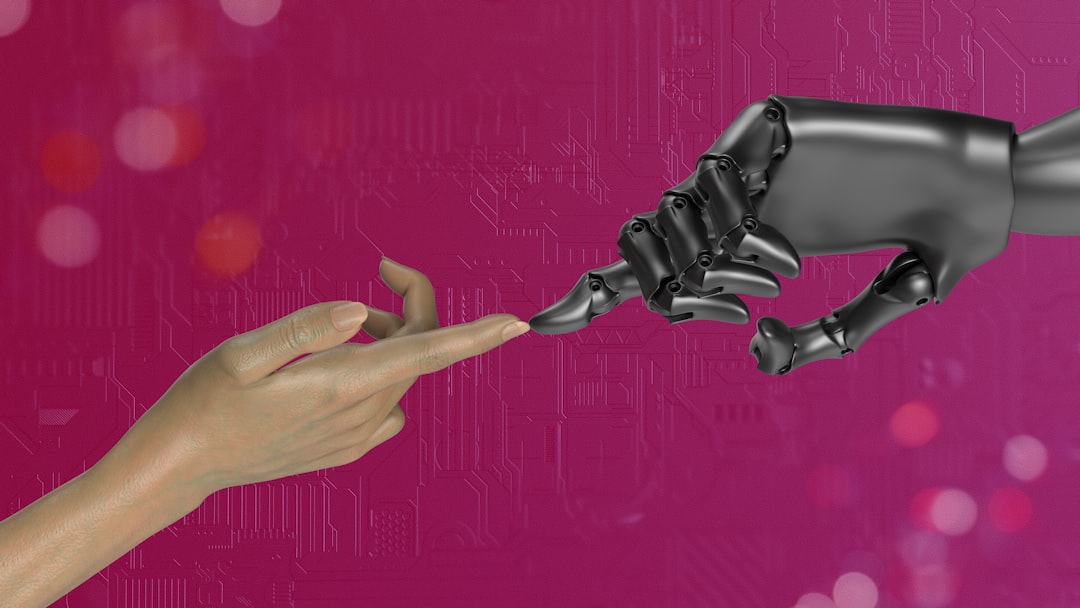
The State v. Miller case showcases how machine learning algorithms are becoming indispensable in identifying manipulated video evidence. The rise of sophisticated digital manipulations, such as deepfakes, presents a significant challenge to the integrity of video evidence in legal proceedings. AI researchers are developing increasingly sophisticated detection methods that leverage artificial intelligence techniques, including convolutional and recurrent neural networks, to scrutinize video data for inconsistencies that could signal manipulation. This continuous improvement in AI's ability to analyze video data emphasizes the need for legal systems to adapt and evolve their processes for authenticating digital evidence. The need to differentiate genuine videos from manipulated ones is becoming ever more crucial in maintaining the integrity of courtroom proceedings in this age of advanced digital technology. Thus, the interaction between AI and legal standards is critical in ensuring fairness and preventing manipulated evidence from unduly influencing legal outcomes.
1. Machine learning algorithms are demonstrating a powerful ability to scrutinize video evidence for manipulation, often uncovering subtle alterations that human eyes might miss. This has significant implications for legal investigations, providing a powerful tool for examining digital evidence presented in court.
2. Cases like State v. Miller highlight the effectiveness of AI in detecting video manipulation. By leveraging techniques like examining inconsistencies in temporal patterns and conducting pixel-level analysis, AI tools can expose evidence tampering and help establish the authenticity of video recordings.
3. The application of AI in legal settings has given rise to automated systems capable of classifying evidence based on authenticity. These systems streamline the initial stages of evidence analysis in trials, saving valuable time and resources for legal teams.
4. Some machine learning models are designed with adaptability in mind, allowing them to quickly learn and recognize new forms of video manipulation. This is crucial in the evolving landscape of digital evidence, where malicious actors continually develop more sophisticated ways to alter media.
5. AI's role in evidence authentication extends beyond video analysis. It's also proving invaluable in analyzing audio recordings. Voice recognition algorithms, for instance, can verify speaker identity and detect subtle changes in tone or pitch that might indicate tampering.
6. The increasing use of AI in evidence verification has prompted discussions about the reliability of traditional forensic methods. It's challenging long-held assumptions about the exclusive role of human experts in evidence analysis, encouraging a reevaluation of the balance between human judgment and algorithmic assessment.
7. As AI becomes more integrated into legal proceedings, ethical questions surrounding accountability and potential biases in algorithmic decision-making arise. It's crucial to establish transparent guidelines for the use of AI in investigations to ensure fairness and mitigate any negative impacts.
8. Large law firms are actively investing in AI technologies for tasks like eDiscovery and case preparation. Some reports suggest that AI could automate up to 30% of legal research, freeing attorneys to focus on complex legal strategy and client interaction.
9. AI's impact on the legal landscape extends beyond evidence analysis and into the realm of document creation. Automated drafting tools are becoming increasingly popular, leading to faster turnaround times and potentially lower costs for routine legal documentation.
10. The continuous development of AI in the legal field raises the need for standardized evidence evaluation procedures. The integration of algorithmic tools necessitates the creation of best practices to ensure consistency, fairness, and reliability in the application of AI during legal proceedings.
7 Critical Ways AI is Transforming Digital Evidence Authentication in Frame-up Cases - Natural Language Processing Tools Track Document Alterations in Federal Cases
Artificial intelligence, particularly natural language processing (NLP), is transforming how legal professionals handle documents, especially in federal cases where evidence integrity is paramount. NLP tools are now adept at detecting alterations in documents, pinpointing inconsistencies and potentially manipulated sections that might otherwise go unnoticed. This ability to analyze language and structure within legal documents enhances accuracy and reliability in document management.
The benefits extend to streamlining the document review process, crucial when dealing with the immense volume of legal papers in federal cases. By automating the identification of patterns, inconsistencies, and critical clauses, AI can help legal teams work more efficiently, reducing the strain on resources and the time spent on manual analysis.
Moreover, there's a growing trend towards integrating human review with AI-driven analysis. This 'human-in-the-loop' approach ensures that potentially sensitive or contextually significant sections are handled carefully, particularly when it comes to redacting sensitive information or understanding nuanced legal language. The combined strength of human oversight and advanced NLP analysis leads to a more reliable and trustworthy system for managing digital evidence, especially in situations where document integrity is critical. Ultimately, the integration of NLP tools helps reinforce the legal system's commitment to accuracy and fairness in a digital age characterized by the increasing sophistication of evidence manipulation.
Natural Language Processing (NLP) tools are increasingly being used to analyze legal documents in federal cases, particularly to detect modifications or inconsistencies. This application of AI has the potential to significantly improve the accuracy and efficiency of legal document management. For instance, by identifying patterns and discrepancies within documents, these tools can help legal professionals more effectively assess risks associated with legal agreements, a crucial aspect of eDiscovery and due diligence.
Moreover, these NLP-driven solutions can streamline the document review process, which can be especially valuable in federal agencies dealing with extensive volumes of legal documents and reports. AI-driven solutions also often incorporate a human-in-the-loop component, allowing human reviewers to verify the output of AI algorithms, particularly for tasks like redacting sensitive information within documents. Beyond simple identification of changes, AI can even leverage techniques like Natural Language Generation (NLG) to provide concise summaries of large datasets or reports, saving time for legal analysts.
An interesting observation is that document lengths in some federal cases, like those related to taxation and economic activity, seem to follow a skewed distribution. This implies that a substantial portion of the documents in these cases may be unusually long or short, a factor that AI tools could help analyze. NLP algorithms, powered by statistical machine learning and deep learning approaches, can extract valuable insights from text data and uncover patterns that might be hard for human reviewers to spot.
The advancement of language models like OpenAI's GPT-3 further shows how AI is being applied to improve text interpretation and understanding in government operations. The integration of NLP with semantic AI technology offers even more powerful applications, enhancing the efficiency of data processing and regulatory response in the public sector.
While the benefits are clear, it's essential to consider the impact of AI implementation, particularly when it comes to costs and ethics. While AI solutions can reduce operational costs for firms, the upfront cost of implementation and maintenance can be substantial. Also, issues like data privacy, intellectual property, and the potential for biased algorithmic outputs raise important questions that require careful consideration and possibly the development of guidelines to ensure fairness and transparency in the use of AI tools for document tracking and analysis in the legal field.
7 Critical Ways AI is Transforming Digital Evidence Authentication in Frame-up Cases - Computer Vision Systems Flag Synthesized Photo Evidence with 98% Accuracy
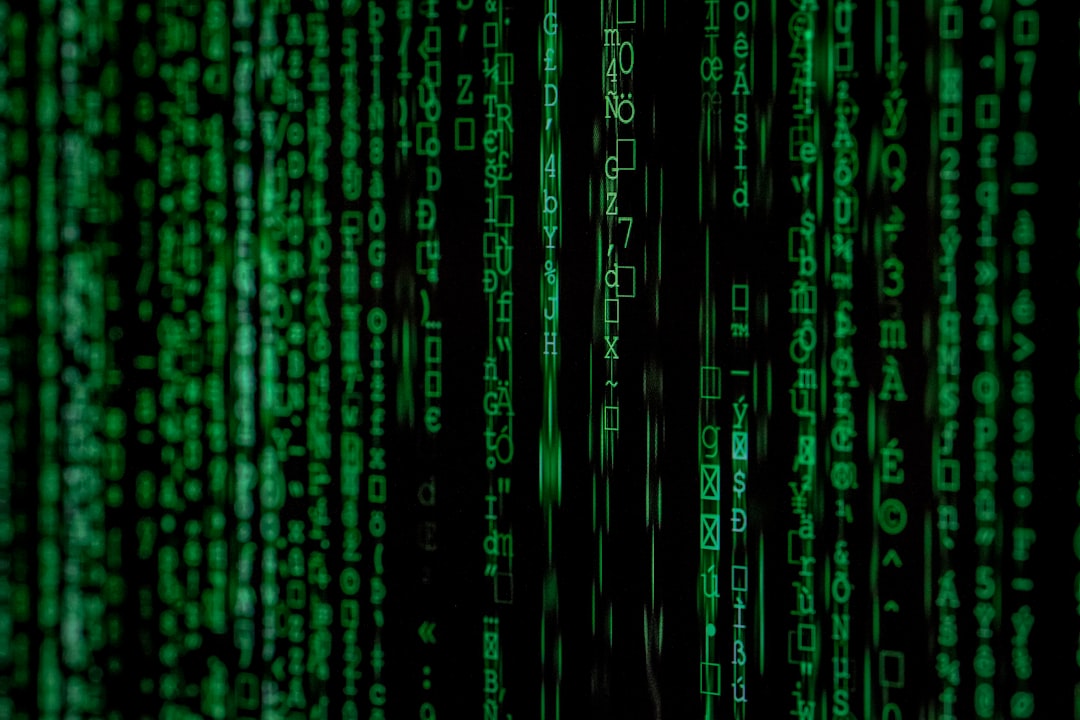
The increasing reliance on digital images in legal matters necessitates robust methods for verifying their authenticity. AI-powered computer vision systems are now achieving remarkable accuracy in identifying manipulated or synthetic photographs, reaching a 98% success rate in some applications. This development has major implications for legal proceedings, as it strengthens the ability of investigators and attorneys to differentiate real images from those that have been digitally altered.
The capability of AI to analyze visual evidence with such precision is transforming how legal professionals handle image-based evidence. It's becoming increasingly crucial to discern genuine photographs from those created using sophisticated image manipulation techniques. By improving the accuracy of image analysis, AI is streamlining the process of authentication, making it easier to expose fraudulent or misleading evidence. This advancement has a direct impact on legal cases, especially those involving allegations of evidence fabrication or frame-ups.
As AI continues to refine its ability to scrutinize visual content, the very nature of how evidence is evaluated in legal settings will evolve. The need for expert human analysis remains, but AI provides a new layer of scrutiny previously unavailable. The implications of this technological shift are far-reaching, ultimately contributing to a more reliable and just system for adjudicating cases involving visual evidence. It highlights how the intersection of AI and law is steadily altering the landscape of legal proceedings, particularly in relation to the growing prevalence of digital evidence.
Computer vision systems are demonstrating a remarkable ability to identify synthesized or manipulated photographic evidence with a 98% accuracy rate. This development is significantly enhancing the process of authenticating digital evidence in legal settings, particularly in cases where the integrity of images is crucial.
These systems leverage advanced AI techniques, specifically deep learning models like convolutional neural networks (CNNs). CNNs are trained on massive datasets of images, allowing them to automatically learn the characteristics of genuine and manipulated images. This learning process enables them to identify subtle inconsistencies or anomalies that may indicate image tampering.
The incorporation of these AI-driven tools into eDiscovery workflows promises to revolutionize how legal teams manage visual evidence. By automating the initial stages of review, lawyers can spend less time on manual analysis and dedicate more effort to the complexities of a case. This can potentially save considerable time and resources.
Furthermore, these systems can be integrated with other AI tools, like natural language processing (NLP) systems that analyze textual evidence. This integration creates a powerful multi-modal approach to evidence verification, allowing for a more comprehensive assessment of the overall evidentiary context.
Another potential application of computer vision in legal settings is cross-referencing images against a database of known manipulated images. This approach adds another layer of security to the authentication process, helping to identify attempts to introduce fabricated evidence.
However, it's crucial to acknowledge that even with their impressive accuracy, these systems are not foolproof. False positives, where a legitimate image is wrongly flagged as manipulated, can occur. Consequently, it's essential for human reviewers to verify the AI's output, ensuring that the integrity of the evidence is never compromised.
As computer vision systems are increasingly used in legal cases, such as the notable State v. Miller case, the body of case law surrounding the admissibility of AI-analyzed evidence is gradually evolving. This growing acceptance is also contributing to the standardization of procedures for introducing such evidence in court.
Moreover, the use of these automated systems holds potential to reduce the overall costs of legal proceedings. Law firms can potentially lessen expenses related to expert witness fees and time-consuming manual image analysis, promoting efficiency in the handling of visual evidence.
However, alongside these advancements come important ethical considerations. The application of computer vision in legal contexts raises issues related to privacy, data protection, and potential biases within the algorithms. It's critical that discussions around the responsible implementation of these AI tools continue to inform policy and ensure ethical and unbiased application within the legal system. This proactive approach is vital as computer vision systems become more prevalent in legal investigations and trials.
7 Critical Ways AI is Transforming Digital Evidence Authentication in Frame-up Cases - Biometric Authentication Validates Digital Evidence Sources Using Multi-Factor Analysis
Biometric authentication is gaining traction as a way to validate the sources of digital evidence, particularly in situations where a person might be falsely accused. This approach combines several different biometric methods, like fingerprint scans, facial recognition, and voice analysis, to create a more reliable system. AI plays a role by utilizing deep learning techniques to improve the accuracy of these methods, which can help counter concerns about evidence tampering. The use of AI in improving biometric authentication may ultimately lead to changes in how legal professionals assess and use digital evidence. Yet, there are potential downsides to relying solely on biometrics for validation. Since this kind of data can't be easily changed or removed, there are heightened security risks if this information gets into the wrong hands. This highlights the need to be aware of biometric data's inherent limitations and to consider how to address them within the legal system to ensure it's used responsibly. As AI continues to evolve and reshape authentication, the legal system will have to keep up with these technological changes, and this includes updating laws and procedures related to digital evidence.
Biometric authentication, aided by AI, is gaining traction in legal settings, particularly for verifying digital evidence. This approach leverages multiple factors like fingerprints, facial recognition, and voice patterns, offering a more robust and accurate method of authentication than traditional single-factor methods. The integration of AI in these systems allows for sophisticated multi-factor analysis, automating some of the processes involved in validating evidence sources. This is becoming increasingly important in fields like eDiscovery where the sheer volume of documents necessitates more efficient methods.
The unique and inherent characteristics of biometric data make it difficult to replicate, thereby enhancing the integrity of digital evidence. This is crucial in situations where evidence manipulation is suspected or where the authenticity of the evidence is paramount. Recent studies suggest that AI-powered biometric systems can significantly reduce the time needed for evidence verification. While this is a welcome development for busy legal teams, it also raises concerns about potential biases or errors in the AI algorithms.
One area of concern is the security of biometric data. While it adds a layer of protection, it is not impervious to compromise. Should the biometric information be breached, it could have serious consequences, highlighting the need for robust security measures. Furthermore, the dynamic nature of AI algorithms allows for the inclusion of user behavior analytics alongside biometric data. This can provide a more holistic view of the evidence handling process, possibly leading to the detection of anomalies that could signal potential fraud.
While the promise of biometric authentication is clear, it also presents ethical considerations. For instance, there are concerns about the implications for privacy and data protection, especially in the context of sensitive legal proceedings. There's also a debate about the potential for AI biases within the algorithms used for authentication, which may lead to inaccurate or unfair outcomes.
Despite these challenges, the integration of AI and biometric authentication offers a path toward more reliable digital evidence verification. The increased redundancy of checks created by these multi-factor methods can contribute to a more robust validation process. However, as legal firms embrace this technology, it becomes crucial to consider whether the current legal framework is sufficient to handle the unique challenges and complexities associated with AI and biometrics in the context of legal practice. This is an evolving area with far-reaching implications for the justice system.
7 Critical Ways AI is Transforming Digital Evidence Authentication in Frame-up Cases - Neural Networks Cross-Reference Evidence Metadata Across District Court Records
Neural networks are being used more and more to compare and contrast the metadata of evidence across different district court records, which is a significant leap forward in how digital evidence is authenticated. These AI systems can analyze massive amounts of metadata to spot inconsistencies and verify the validity of evidence. This is crucial to making sure that digital evidence is allowed in court. This capability greatly improves how legal professionals verify digital records, helping to address concerns about evidence manipulation and deceit. Furthermore, the growing ability of these neural network algorithms can make processes within law firms more effective, cutting down on time spent on repetitive manual checks. Ultimately, these types of innovations show the significant potential of AI to protect the integrity of digital evidence in today's legal world, where digital evidence is increasingly important.
1. Neural networks are being explored to sift through the massive amounts of data within district court records, aiming to spot inconsistencies and patterns hidden within the metadata of evidence. This has the potential to streamline the authentication of digital evidence, possibly saving lawyers a significant amount of time in the pre-trial phase.
2. Deep learning models are being employed in legal research to help cross-reference cases and related legal statutes in real-time. This might reveal previously unnoticed information that could affect the outcome of a legal case. It's like having an extra researcher always on the job, searching for the most relevant precedents.
3. AI systems trained on evidence metadata are being developed to autonomously identify unusual data points in legal files. If something seems out of place, it can trigger a more thorough review by a human investigator. This potentially improves the quality of judicial decisions by flagging potential issues early on.
4. In many places, neural networks are being integrated into eDiscovery procedures, aiming to cut down on the time spent reviewing documents by up to half. This freeing up of time and resources could shift the focus of legal teams to more complex strategic legal tasks.
5. Sophisticated AI algorithms are being experimented with for cross-referencing evidence metadata to ensure its accuracy, which is crucial for evidence to be admitted into a court proceeding. Maintaining accuracy in metadata is important, as it can be used to verify when and how digital evidence was created or modified.
6. AI tools in legal settings are being examined to track changes in how evidence is documented over time. This ability to track changes may be useful to construct a strong legal argument, particularly in situations where someone might be falsely accused.
7. Ongoing training of neural networks on district court decisions can improve their ability to predict future outcomes in similar cases, based on the rulings in previous cases with a similar context. This can help legal professionals strategize for their cases and help anticipate possible outcomes.
8. Automating the analysis of metadata across district court records using AI can help reveal possible patterns of judicial bias. It is important to address potential issues of fairness in the legal system.
9. AI systems can potentially analyze evidence metadata to discover previously unknown connections between defendants and other cases, potentially surfacing broader problems in the system that may need to be fixed.
10. As the reliability of AI-driven metadata verification improves, questions regarding the responsibility of these AI systems arise. It's important to consider who is liable if an AI-generated conclusion ends up influencing a legal decision.
7 Critical Ways AI is Transforming Digital Evidence Authentication in Frame-up Cases - Pattern Recognition Technology Maps Evidence Tampering in Financial Fraud Cases
AI-powered pattern recognition is becoming increasingly important in legal proceedings, especially for identifying potential evidence tampering in cases of financial fraud. These advanced algorithms can analyze large amounts of data, uncovering subtle inconsistencies that might indicate manipulation. Financial fraud schemes are constantly evolving, so the AI models used to detect them must also be continuously improved. This continuous development is crucial as we see a growing reliance on digital evidence in legal matters. By integrating AI into legal procedures, we can bolster defenses against attempts to compromise the integrity of evidence. This technology has the potential to significantly change how financial fraud cases are investigated and prosecuted, strengthening the pursuit of justice in our increasingly digital society. The use of pattern recognition for evidence analysis may help ensure that the legal system remains effective at combating complex and rapidly evolving forms of financial crime.
AI's capacity for pattern recognition is increasingly valuable in financial fraud cases, particularly in identifying evidence tampering. For example, AI can analyze email and messaging patterns, looking for unusual communication flows or language that might signal manipulation. This is particularly useful when there's a suspicion of collusion or fabricated evidence. The constant evolution of fraud tactics necessitates AI models that continuously learn and adapt to new schemes, highlighting the need for ongoing development in this area. Major financial institutions, such as JP Morgan Chase, are actively engaged in research and development, striving to enhance their AI capabilities for fraud detection and prevention.
AI also plays a key role in the growing reliance on digital evidence within law enforcement. Experts recognize that AI can be an extremely powerful tool for fraud investigators, helping them to sift through large volumes of data and uncover hidden patterns that might be missed by human analysts. Generative AI approaches are also showing great promise in real-time fraud detection, allowing financial institutions to react quickly to potentially fraudulent activities. However, the increased reliance on AI in this domain brings forth a need for robust mechanisms to verify the integrity of the AI's output, especially when it comes to identifying evidence tampering.
While AI's capabilities are powerful, the increasing use of digital currencies and the rapid evolution of financial technology present challenges for AI tools. These systems need to be continuously adapted to meet regulatory guidelines and ensure compliance with evolving legal standards. Furthermore, AI algorithms must be evaluated for bias and fairness, especially when they're used to make decisions that could potentially impact a person's legal standing. There's a growing recognition that regular scrutiny of the plausibility of evidence tampering is essential, even when there are no obvious signs of manipulation, and this area benefits greatly from AI's ability to detect hidden patterns. The complexity of digital forensic evidence necessitates sophisticated AI-driven approaches to improve pattern recognition, highlighting its critical role in this demanding area of investigation.